Business Challenges: 10x Business Expansion, 10x AIOps Scaling
Artificial Intelligence for IT Operations (AIOps) takes artificial intelligence as the core to realize automatic, efficient, and low-cost IT operation and maintenance management. Through machine learning algorithms, big data analysis, feature extraction and rule-based modelling, AI-powered operation and maintenance functions such as intelligent monitoring, intelligent fault analysis and intelligent alarm are now possible. In recent years, with the rapid expansion of the scale of enterprise data centers, AIOps has become more and more important for large-scale online applications and has been implemented in many practical scenarios.
The AIOps in a large financial institution is used to monitor the abnormal operations of the system of online services. For example, when the abnormal jitter of the system transaction volume is monitored, it is necessary to decide whether to start the operation warning and let the operators investigate the issue. Due to the sharp increase of online business in recent years, the current AIOps system is overwhelmed and there is a strong demand for capacity expansion and upgrading. The organization expects that its throughput can be expanded 10 times a year on the premise that the latency performance remains unchanged. However, if the capacity is expanded on the original technical solution, customers need to expand their machine resources 10 times a year, which is extremely expensive.
The AIOps of this customer is built based on a set of complex machine learning algorithms. Each specific monitoring object will do multiple sets of parallel feature extraction and model prediction. Finally, it will go through a rule-based system to decide whether to alarm the administrators. Since the overall resource consumption bottleneck of the intelligent operation and maintenance system is on its feature computing platform, the organization expects to build a low-cost capacity upgrade solution based on OpenMLDB.
Solution: Efficient and Low-Cost Upgrade of AIOps based on OpenMLDB
In the process of in-depth cooperation between the OpenMLDB team and the customers of the financial institution, the bottleneck of the AIOps is identified. The customer's existing feature computing and management platform are built based on Redis. Although Redis has excellent in-memory data access efficiency, it is not optimized for feature computing. Especially when complex feature computing logic such as time-window is involved, Redis needs to consume a lot of computing and memory resources to achieve the expected outcomes. The difference is that OpenMLDB provides a full-stack feature engineering platform. Based on this analysis, both teams cooperated to replace the original Redis with the full stack FeatureOps capability of OpenMLDB to realize the optimization of feature computing.
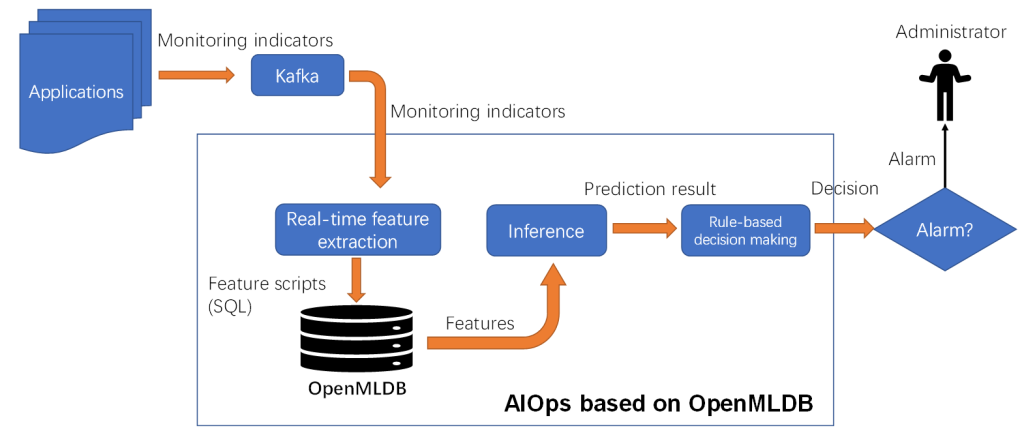
Figure 1 shows the overall system design of the AIOps based on OpenMLDB. The system monitoring indicators are collected through Kafka and then fed into the AIOps platform. For each monitoring object, the platform first extracts the useful feature information in real-time based on OpenMLDB, then obtains the prediction results through model inference, and finally makes rule-based decisions based on the prediction results of multiple sets of parallel models to finally determine whether to report the alarm to the administrator.
Business Value: Significant TCO Reduction for AIOps
After the customer replaced Redis with OpenMLDB as its feature computing platform for AIOps, the main business benefits obtained are as follows:
- Resource consumption reduced significantly. The existing business of the customer's AIOps has reduced the CPU resource consumption by 3 times and the in-memory consumption by 2 times.
- Low-cost business expansion. The customer aims to achieve 10 times of business expansion every year. The original Redis based scheme needs to increase 10 times of physical resources every year to meet business needs. After using OpenMLDB, the target capacity expansion can be achieved by only increasing physical resources by about 5 times, saving nearly twice the total cost.
- OpenMLDB-based AIOps further enhances business effectiveness. Because OpenMLDB provides a computing engine optimized for feature computing and the SQL-centric experience, customers can more flexibly implement feature extraction scripts. In the past, Redis based feature computing development brought many inconveniences and restrictions. After using OpenMLDB, its feature computing functionality has been further enhanced, which can implement more effective feature extraction logic and improve the overall effectiveness of AIOps.