Why OpenMLDB
OpenMLDB Provides a Full-Stack FeatureOps Solution for Production
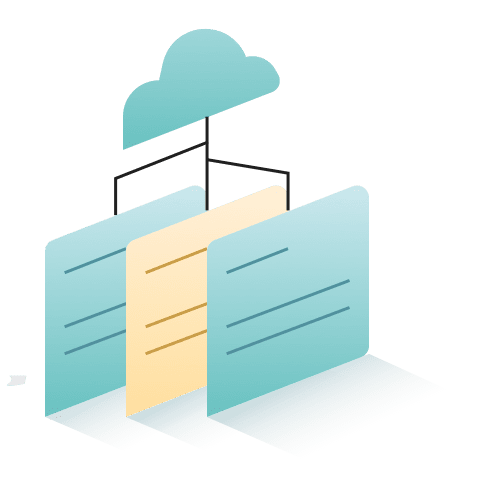
SQL-Centric Development and Management
Feature extraction script development, deployment, and maintenance are all based on SQL with great ease of use.
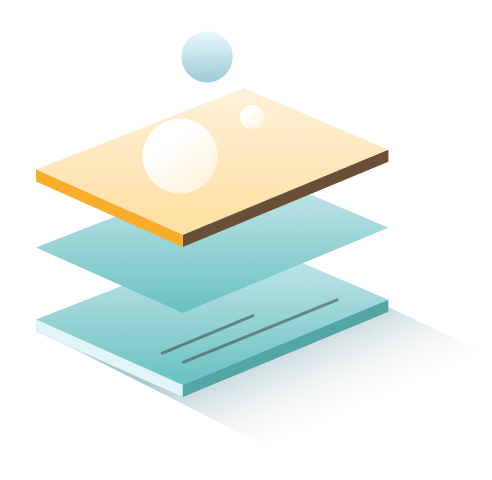
The Unified Online-Offline Execution Engine
Offline and real-time online feature extraction use a unified execution engine, thus online-offline consistency is inherently guaranteed.
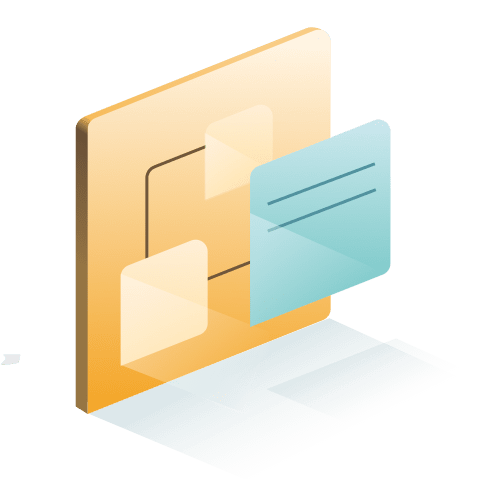
Customized Optimization for Feature Extraction
Offline feature extraction is performed based on a tailored Spark version that is particularly optimized for batch-based feature processing. Online feature extraction provides tens of milliseconds latency under high throughput pressure, which fully meets the online performance requirements.
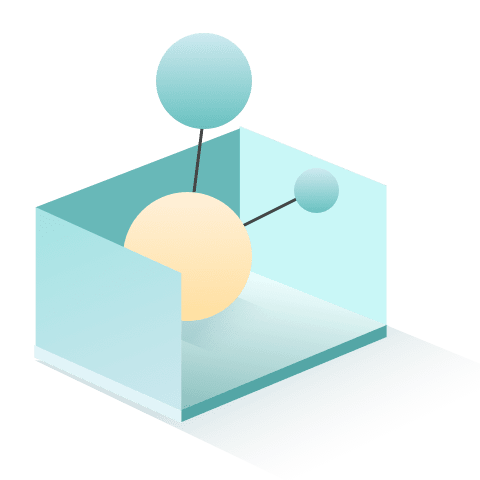
Production-Ready
OpenMLDB has been implementing important production features for large-scale applications, including fault recovery, high availability, seamless scale-out, smooth upgrade, monitoring, heterogeneous memory support, and so on.
Use Cases
OpenMLDB is Driving the AI Transformation for Enterprises
more
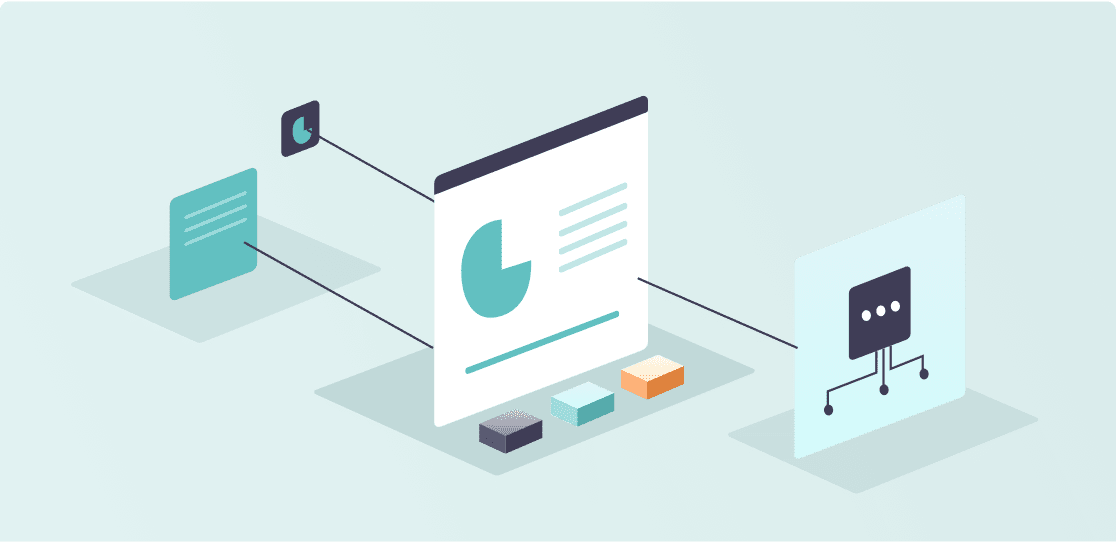
OpenMLDB Hive Connector, connecting the data warehouse and feature engineering
The introduction of OpenMLDB Hive Connector allows you to continues to build an offline data ecosystem. This article is expected to build a more comprehensive ecosystem, which can lower the threshold of users while attracting more users and the development has solved the issue of being unable to connect easily and using Hive data sources in OpenMLDB.
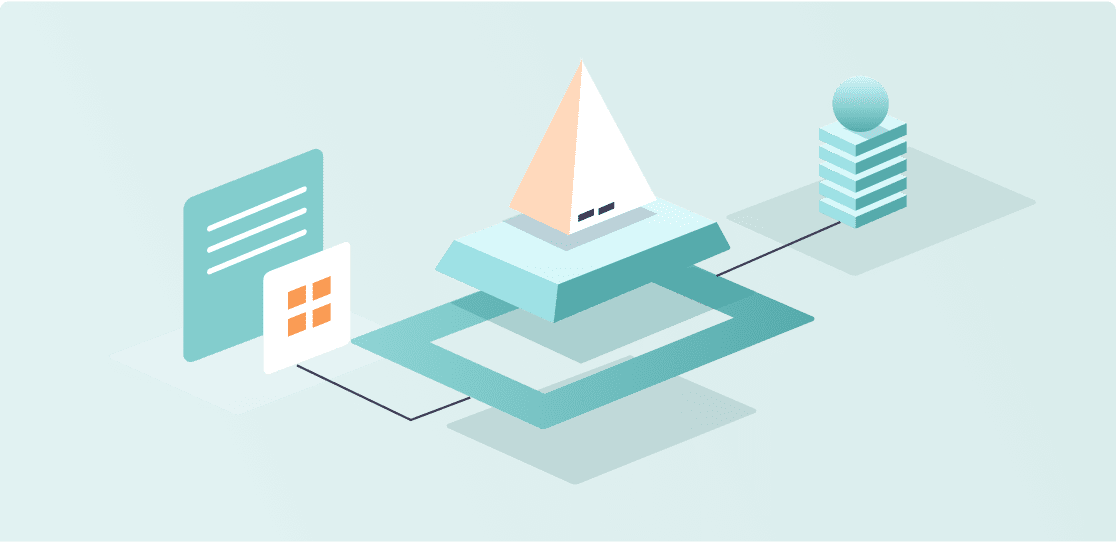
OpenMLDB Pulsar Connector: Efficiently Connect Real-Time Data to Feature Engineering
One of the key points for the engineering implementation of artificial intelligence is to solve the problems of real-time batch estimation and real-time model updating of real business scenarios. Better and faster transformation of online real-time data into AI usable features will accelerate the efficiency and effect of AI application landing. To this end, OpenMLDB and Apache Pulsar jointly launched the OpenMLDB Pulsar Connector to achieve stable streaming integration and provide a clear path to efficiently get through real-time data to feature engineering.
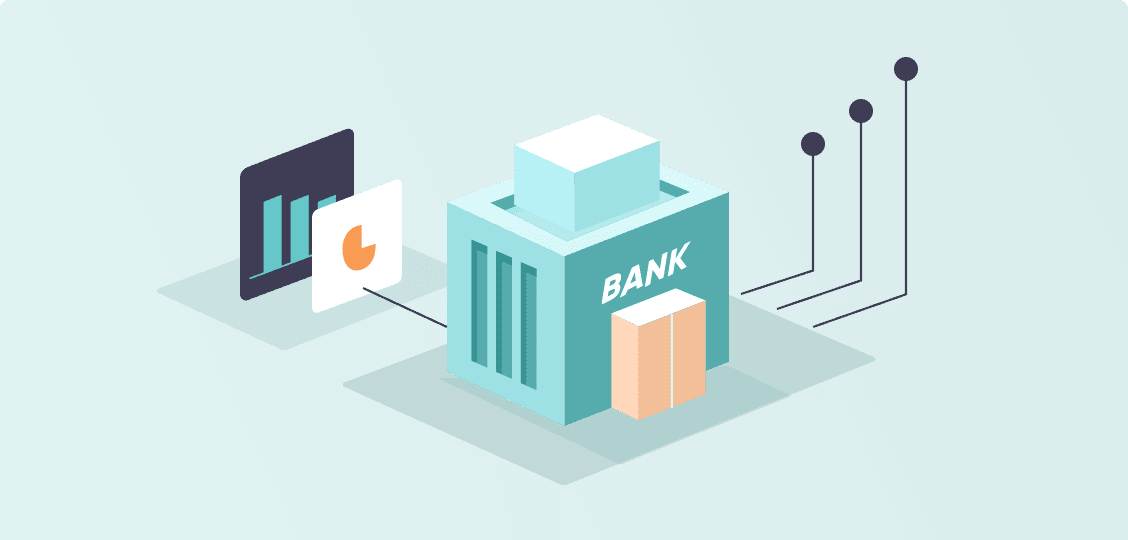
Akulaku: Real-Time Feature Extraction for AI-Powered Risk Control
In financial technology scenarios, OpenMLDB not only doubles the team's human efficiency and saves millions of costs, but also is the only solution with linear scale compared with Spark and Flink.
OpenMLDB introduction video